Articulated Human Pose Estimation
Mykhaylo Andriluka & Leonid Pishchulin
Articulated Human Pose Estimation
Human body pose contains a wealth of information about a person’s intention, attitude and internal state. The focus of our research is to estimate body pose in realistic conditions such as images and videos found on YouTube or captured with a mobile phone. We envision that developed approaches will become building blocks in such applications as activity recognition, markerless motion capture and augmented reality.
We build on the recent advances in hierarchical image representations with convolutional neural networks (CNN) and explore two novel research directions: joint estimation of poses of multiple people and 3D human pose estimation from only a few mobile cameras.
Detection and pose estimation in multi-person scenes
We propose an approach that jointly solves the tasks of articulated person detection and pose estimation. Our approach infers the number of persons in a scene, identifies occluded body parts, and disambiguates the body parts of different people in close proximity of each other [ see figure ]. Our formulation is based on partitioning and labeling a set of body part hypotheses generated with a CNN-based body part detector. The partitioning is accomplished by solving an integer-linear program that resembles correlation clustering approaches previously proposed for image and video segmentation. One of the advantages of our formulation is that it implicitly performs non-maximum suppression, removing spurious body part detections in the background and merging multiple correct detections corresponding to the same person. We evaluate our approach on standard benchmarks showing its advantages over previously proposed strategies that operated by first detecting the people and then independently estimating their body poses.

Multi-view 3D human pose estimation
We propose a novel method for the accurate marker-less capture of articulated skeleton motion of several subjects in general scenes, indoors and outdoors, even from input filmed with as few as two cameras. Our approach unites a discriminative image-based joint detection method with a model-based generative motion tracking algorithm through a combined pose optimization energy. The discriminative part-based pose detection method, implemented using convolutional neural networks, estimates unary potentials for each joint of a kinematic skeleton model. These unary potentials are used to probabilistically extract pose constraints for tracking by using weighted sampling from a pose posterior guided by the model. In the final energy, these constraints are combined with an appearance-based modelto image similarity term. Poses can be computed very efficiently using iterative local optimization, as CNN detection is fast, and our formulation yields a combined pose estimation energy with analytic derivatives. In combination, this enables us to track fully articulated joint angles at state-of-the-art accuracy and temporal stability with only very few cameras.

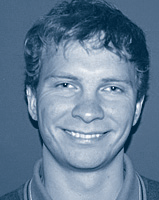
Mykhaylo Andriluka
DEPT. 2 Computer Vision and Multimodal Computing
Phone +49 681 9325-2119
Email andriluk@mpi-inf.mpg.de
Leonid Pishchulin
DEPT. 2 Computer Vision and Multimodal Computing
Phone +49 681 9325-1208
Email leonid@mpi-inf.mpg.de