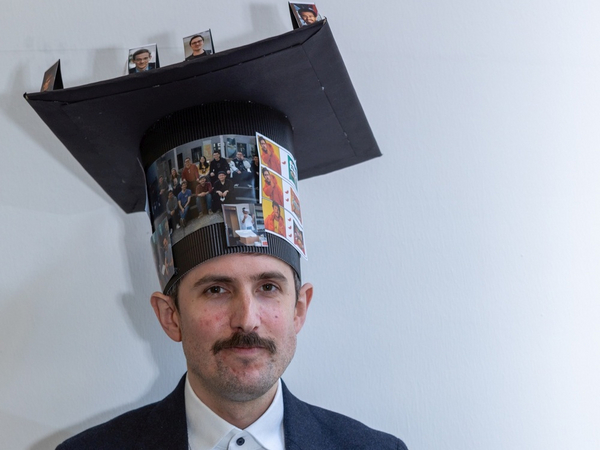
Max Losch with mortarboard.
On April 11, 2025, Max Losch successfully defended his PhD thesis entitled “Improving Trustworthiness of Deep Learning via Inspectable and Robust Representations”. Max Losch joined Saarland University and the Max Planck Institute for Informatics in 2017 as a doctoral candidate under the supervision of Professor Bernt Schiele. He was a member of both the Saarbrücken Graduate School of Computer Science and the International Max Planck Research School. His doctoral degree was awarded by Saarland University.
The abstract of his Thesis:
An increasing number of Deep Learning systems are applied in the real world and have potential impact on people’s lives: autonomous cars, assisted medical diagnosis or social scoring. Attributable to training increasingly complex models on increasingly large datasets, these applications have become useful since they are trained to be accurate prediction machines. Typically, this is achieved by optimizing for accuracy only while disregarding two critical weak points of deep learning persisting since its inception. Firstly, the complexity of used models render it difficult to explain and understand causes for incorrect predictions – coined as black box property. Secondly, models are susceptible to adversarial examples – slight input perturbations otherwise imperceptible to humans – that can result in dramatic changes in predictions. While mitigation approaches exist, these are often expensive to train and hence are not deployed by default in practice. Both issues reduce the trustworthiness of deep learning and could dampen further adoption for real world problems. In this thesis defense, I discuss mitigations for both issues in two parts. In the first part, I discuss our proposed Semantic Bottlenecks that explicitly align intermediate representations to human meaningful concepts like feet, leg, wood, etc. while reducing dimensionality to address the black-box issue and show that these bottlenecks can be useful for error analysis. In the second part, I discuss two ways to mitigate the risk to adversarial examples with a focus on reducing the computational over-head of conventionally used adversarial training:
(i) training on data subsets and
(ii) utilize Lipschitz bounds to enable certification.